If you’ve ever wondered how to earn money by promoting products online, affiliate marketing might be your answer. This guide cuts through the noise to deliver straightforward insights into establishing fruitful affiliate relationships, choosing your niche, and developing effective strategies. Without any fluff, expect to receive the knowledge you need to start or optimize your affiliate marketing journey for better results.
Key Takeaways
- Affiliate marketing revolves around promoting products or services for commissions, and to succeed, one must understand the roles of affiliates, merchants, networks, and maintain transparency.
- In affiliate marketing, choose a niche you’re passionate about and that has demand, select programs with favorable commission structures, use SEO and create high-quality content to drive traffic and conversions.
- Tracking performance with tools like Refersion and Tracknow can refine your affiliate strategy, and adapting to changes and challenges is critical for long-term success and building trust with your audience.
Understanding Affiliate Marketing
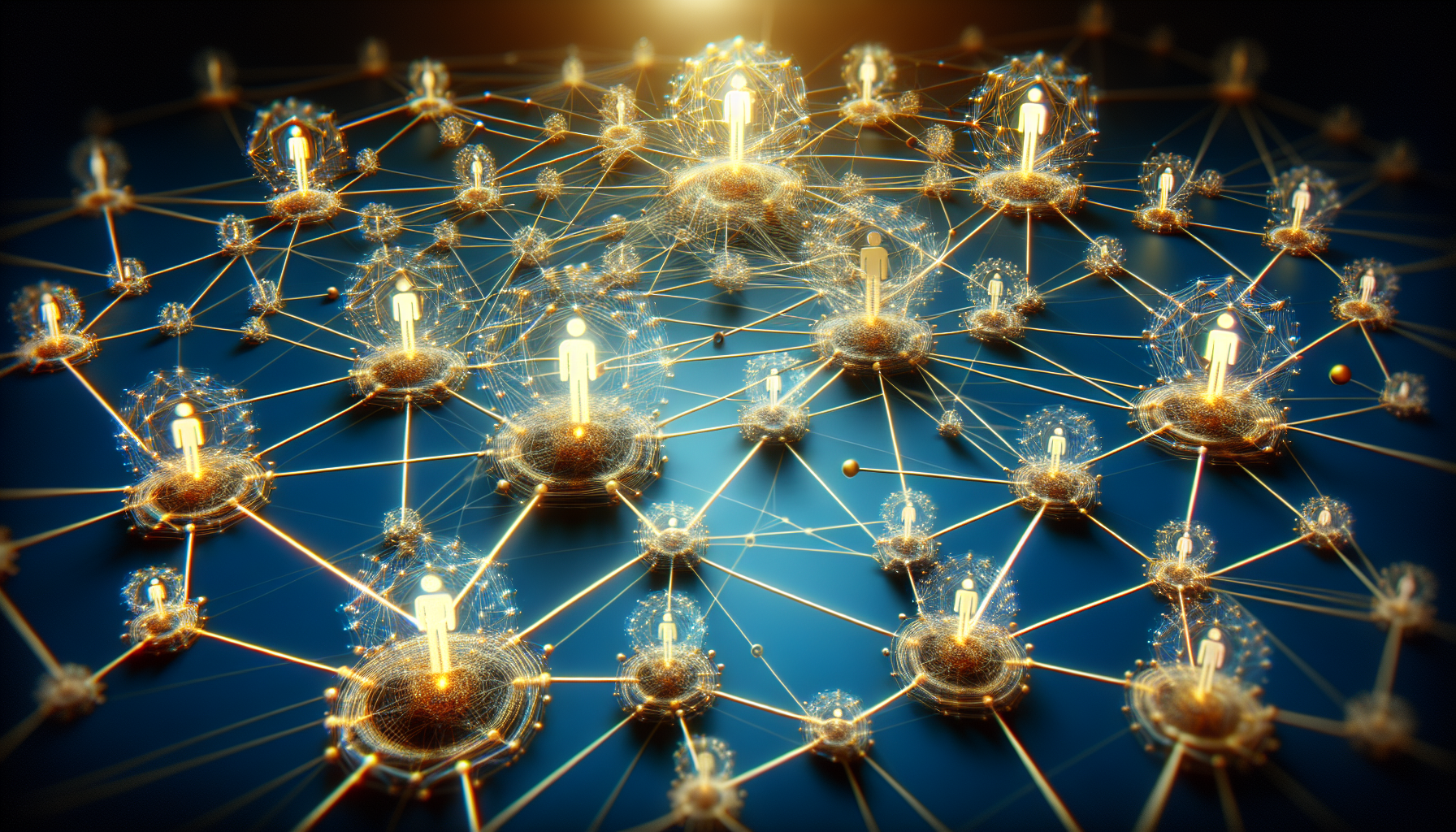
Affiliate marketing is a fascinating world where individuals, known as affiliates, promote a merchant’s products or services for a commission. This industry has four core players: the merchant or ‘retailer’, the affiliate or ‘publisher’, the affiliate network handling offers and payments, and ultimately, the customer. This strategic model offers a win-win situation for both merchants and affiliate marketing partners by increasing brand awareness, driving targeted traffic, and rewarding sales or leads with commissions. In this ecosystem, involved affiliate marketing work plays a crucial role in the success of online businesses.
In the digital landscape, affiliate marketing holds a significant place, often integrating with other marketing methods, contributing to a brand’s online presence, and fuelling revenue streams. By optimizing your affiliate marketing efforts, you can further enhance your brand’s success in this domain and prove that affiliate marketing works.
The Role of Affiliates
Affiliates are the backbone of this model, using personalized marketing techniques to promote merchant’s products or services to their audience. Their reward? Performance-based commissions when a consumer clicks on the affiliate’s link and completes a purchase or other desired actions. However, affiliates need to maintain transparency by disclosing their relationship with the merchant and any affiliate links, in accordance with regulations like those set by the FTC. To achieve this, they often collaborate with affiliate networks that help ensure compliance and facilitate partnerships.
Affiliates must also comprehend the commission structure thoroughly and adhere to the merchant’s terms and conditions.
The Role of Merchants
Merchants play a pivotal role in the affiliate marketing ecosystem. They:
- Create products or services
- Set up the commission and payment systems
- Launch affiliate programs
- Select suitable affiliates
- Ensure ongoing support for them
A clear blueprint for the affiliate marketing campaign is necessary for merchants to ensure smooth sailing, encompassing goals, objectives, financial management, and strategies for strong affiliate relations. Also, having clear terms of service and privacy policies is paramount to avoid conflicts with affiliates.
Merchants also provide affiliates with promotional materials, affiliate links, and sometimes, dedicated affiliate support teams. The merchant’s supportive role necessitates regular communication with affiliates about new products and promotional campaigns.
Choosing the Right Niche for Your Affiliate Marketing Business
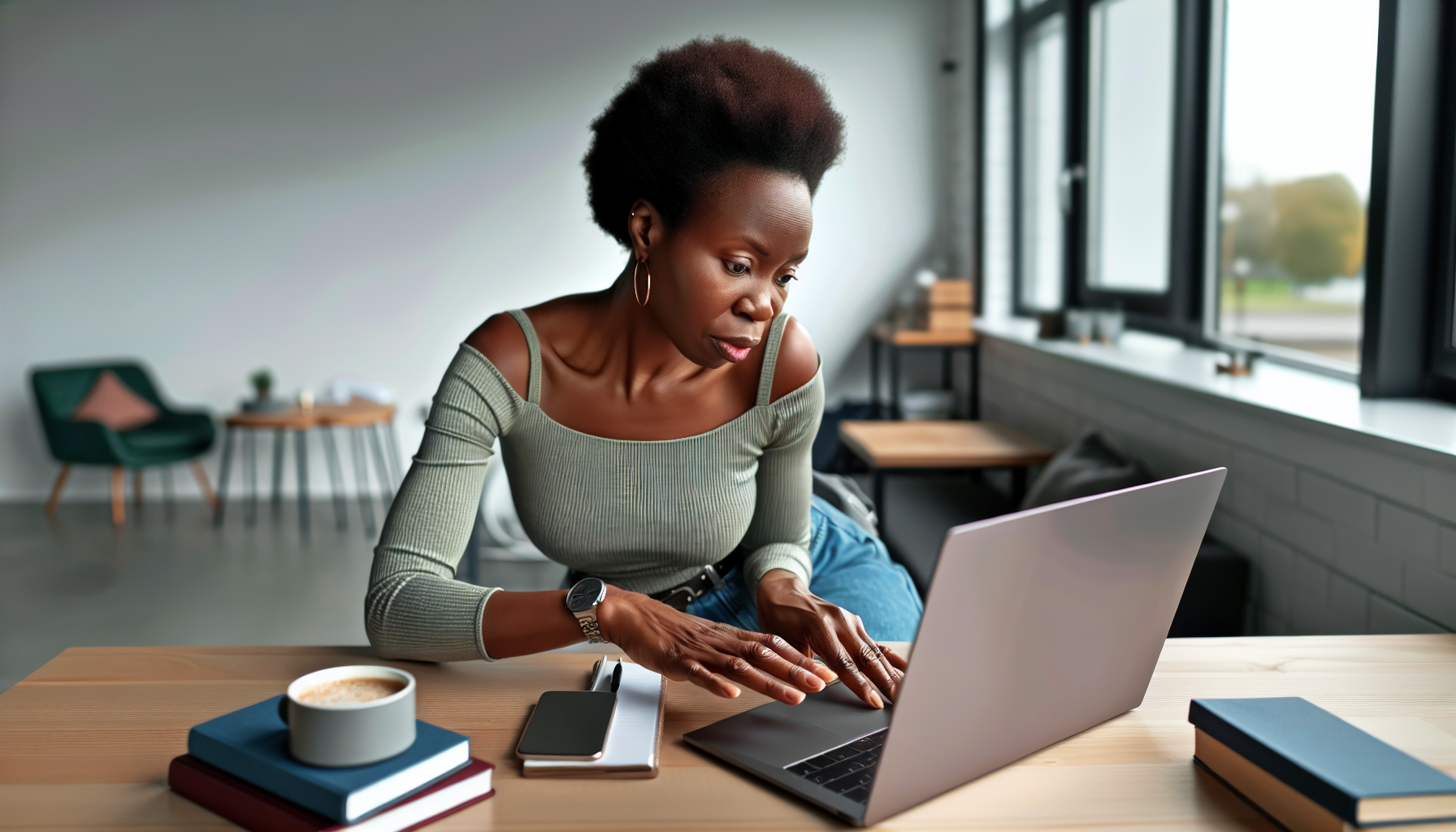
Choosing a niche that aligns with one’s passion increases the enjoyment and likelihood of sustained effort towards successful affiliate marketing. Your personal interest in a niche results in more authentic content, establishing trust and expertise with your target audience. However, striking a balance between passion and profit is crucial by opting for niches with enough demand to guarantee worthwhile returns. Often, niches with high demand but low competition can lead to higher returns.
Understanding the competition provides insights into successful marketing strategies and possible market gaps to exploit. And finally, choosing products within a niche that have high conversion rates and commission percentages can significantly impact your affiliate earnings.
Selecting the Best Affiliate Programs and Networks
Selecting the appropriate affiliate programs and networks is a critical step once you’ve identified your niche. Commission structures in affiliate programs vary, including:
- Flat rates
- Percentage commissions
- Tiered structures
- Revenue Sharing
- Cost Per Action (CPA)
- Pay-per-click (PPC)
- Pay-per-acquisition (PPA)
- Cost-per-lead (CPL)
- Pay-per-impression (PPI)
It’s advisable to assess the Customer Lifetime Value (CLV) when setting affiliate commission rates to guarantee long-term sustainability and profitability.
Clearly defined commission conditions help affiliates understand eligibility for earnings, including specifics surrounding customer product returns within a period. The suitability of an affiliate program also depends on the alignment with the affiliate’s audience, brand, and expertise, and whether the program meets their income goals.
Building an Effective Affiliate Marketing Strategy
Your success hinges on having a robust affiliate marketing strategy. This includes creating high-quality content, optimizing for search engines, and engaging with the audience as part of your marketing efforts to build trust and increase conversions.
Content Creation
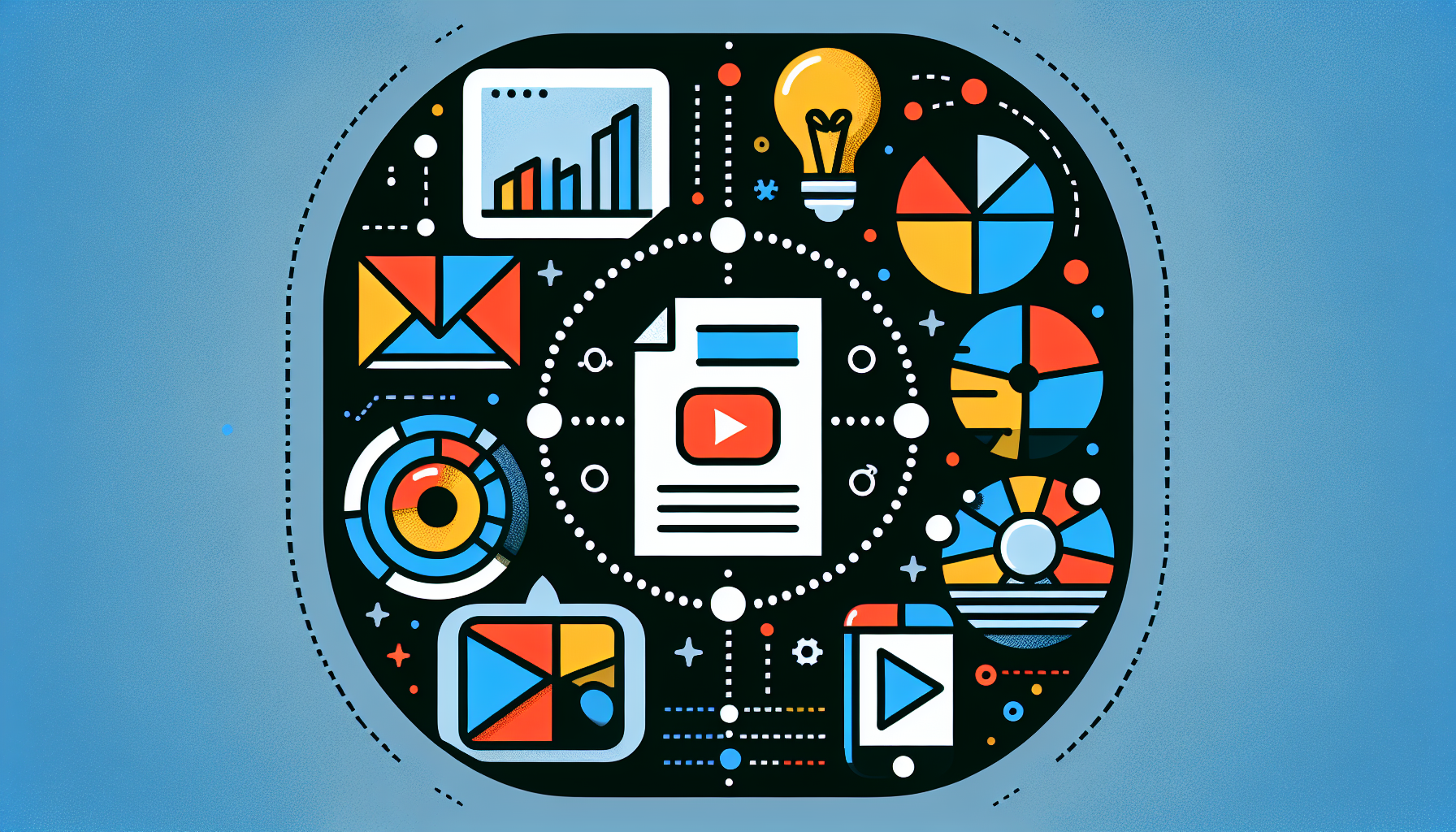
In the world of affiliate marketing, content is king. To succeed, you need to create unique and high-quality content, which drives traffic, builds trust and brand image, and increases conversions. Diversifying your content through:
- visuals
- interactive elements
- blogging
- leveraging coupons and special deals
- including different content types like tutorials and product reviews
enhances user engagement and the overall user experience.
Ensuring your content stays relevant by aligning it with current trends and publishing authoritative materials appeals to your audience.
Search Engine Optimization (SEO)
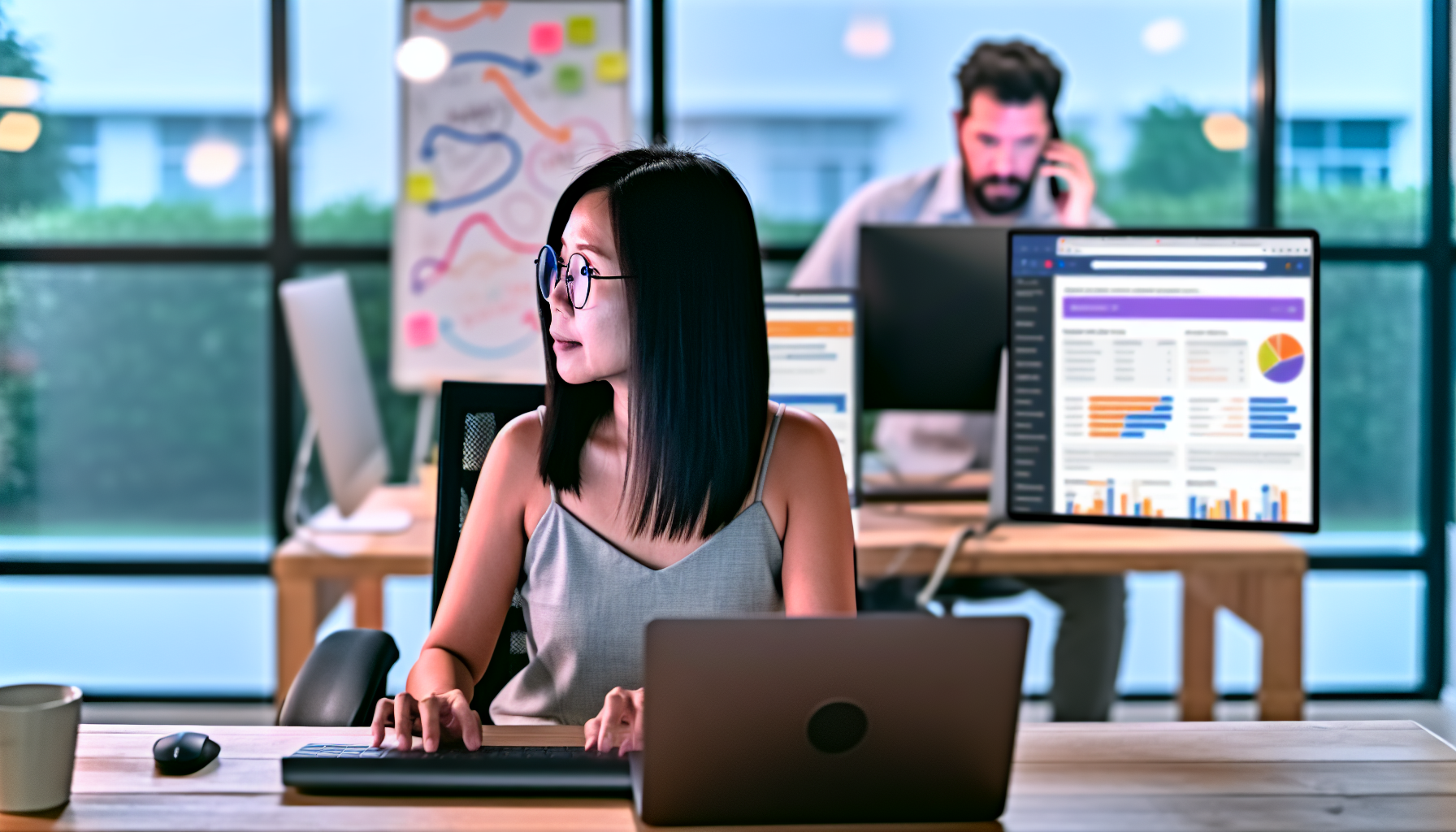
Another integral component of your affiliate marketing strategy is Search Engine Optimization (SEO). SEO increases your website’s visibility on search engines, driving organic traffic, and increasing visibility for your affiliate content. An effective SEO strategy includes:
- Having a keyword-rich domain name
- Employing technical SEO
- Optimizing for voice search
- Creating link-worthy long-form content to strengthen search engine visibility.
For SEO, it’s essential to maintain a consistent schedule of high-quality content updates and strategically incorporate affiliate links in the content to enhance transparency and improve search engine rankings.
Audience Engagement
Engaging with your audience is one of the most effective ways to build trust, which increases the likelihood of them making a purchase from your affiliate links. Guest posting with original, valuable content on niche sites can secure traffic and credibility. Interviewing the founder of an affiliate product builds excitement, trust, and provides a human element to the product being promoted.
Email marketing allows you to connect with your audience and indirectly promote products through high-value content. Regular email updates keep your audience informed and engaged with your content and promotions. Performing random acts of kindness, such as replying to comments and answering questions, help build deeper and more meaningful relationships with your audience.
Utilizing Multiple Marketing Channels
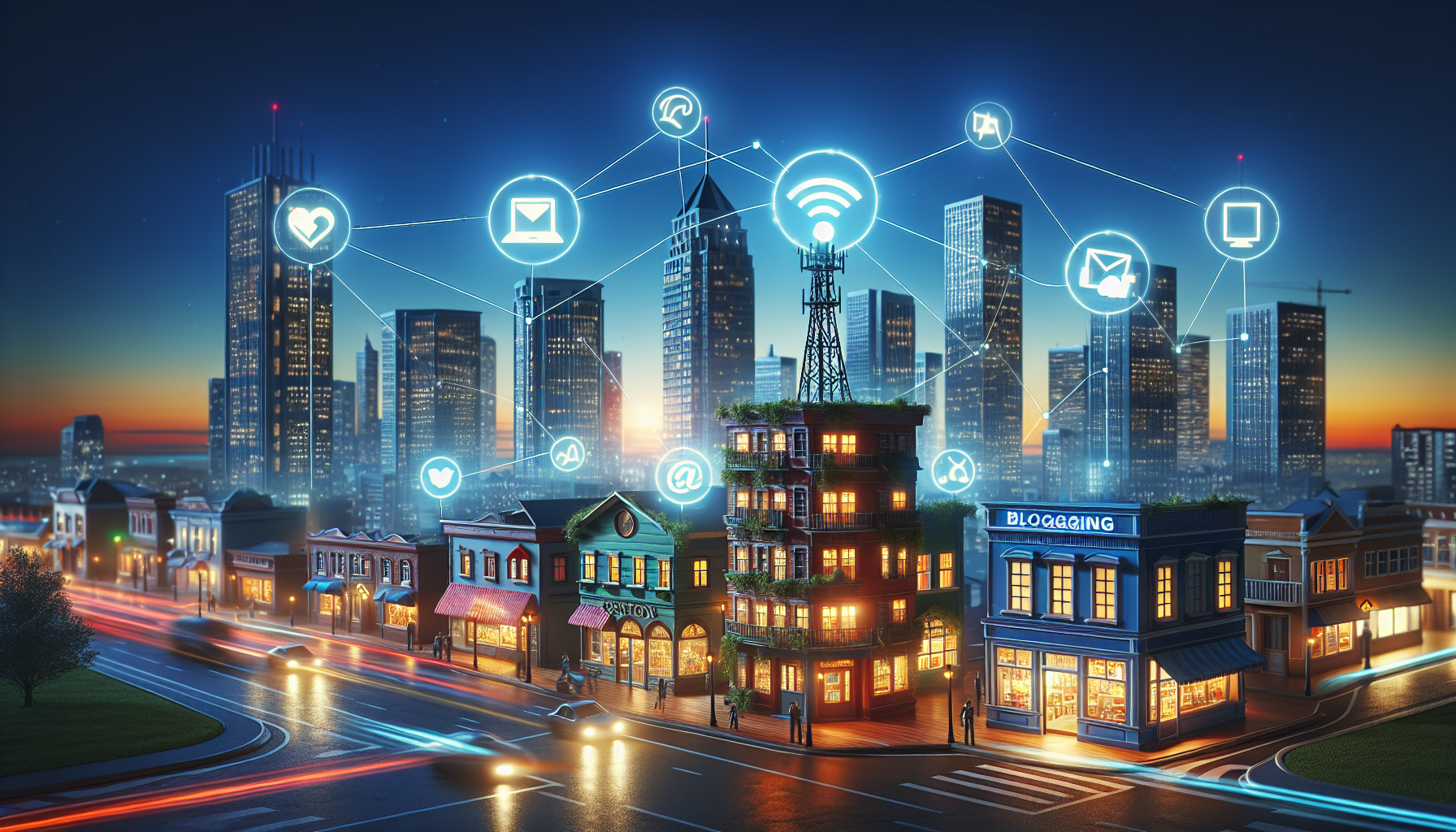
Leveraging a variety of marketing channels can notably amplify your visibility and conversions. Instagram, with its visual content, is particularly effective for affiliate promotion. You can make use of:
- Instagram Stories
- Reels
- Instagram Live sessions
- The ‘link in bio’ feature
To display and share your affiliate link, increasing the likelihood of conversions.
Bloggers can implement affiliate marketing through diverse content such as product reviews, comparisons, and guide posts with strategically placed affiliate links for optimal conversion. Video content, especially product demonstrations, can boost trust and conversions, while webinars allow for a personal connection that can drive affiliate sales.
Developing an email list and engaging in brand partnerships can significantly increase conversion rates for affiliates, offering multiple channels to share affiliate links with audiences.
Tracking and Analyzing Your Affiliate Marketing Performance
It is indispensable to track and analyze your affiliate marketing performance to evaluate the success of your campaigns and make improvements based on data-driven decisions. Merchants use affiliate marketing software to automate and streamline the management of their affiliate marketing program and track affiliate performance indicators such as clicks, page visits, and earned commissions.
Affiliate campaign management tools like Refersion and Tracknow integrate with e-commerce platforms and feature comprehensive analytics to manage campaigns and commissions efficiently.
Overcoming Common Affiliate Marketing Challenges
As with any business venture, affiliate marketing presents its unique challenges. One of the keys to success is building trust and authenticity, which can be achieved by promoting products you’ve personally used or that come highly recommended from trusted sources. Understanding the products and selecting ones that align with your audience’s needs can avoid confusion and reinforce your authority within your niche. Marketers should be prepared to adapt to changes, such as company management shifts, and learn from setbacks to optimize future affiliate campaigns.
Building rapport involves supporting the audience with detailed content and answers to their questions, reinforcing their comfort and security in their investments.
Case Studies: Successful Affiliate Marketers
Successful affiliate marketers tailor their affiliate marketing strategies to their specific niches, using tactics best suited to their target audience and product type. Here are some examples:
- Hobotech reviews high-ticket electricity-related products on YouTube
- Scotty Kilmer focuses on vehicle repair tutorials to earn affiliate income through product recommendations
- RTINGS.com and The Barbecue Lab combine website content with a YouTube presence for maximum visibility
- Bank My Cell implements custom widgets and PR to boost trade-ins and backlink growth
These successful case studies established topical authority, dominated keyword rankings, amassed a significant number of subscribers, and generated considerable affiliate income.
Tips for Long-Term Affiliate Marketing Success
Building a relationship with the audience and consistently delivering value through recommendations are key to achieving long-term success in affiliate marketing. Incentivizing customers through bonuses, such as offering one-on-one sessions, can encourage purchases and sign-ups through affiliate links, fostering customer loyalty.
Affiliate marketers should stay updated on strategies to maximize the use of time and resources to earn more affiliate income. To start affiliate marketing, setting small beginnings with achievable milestones and celebrating successes serve as a motivator, facilitating the scaling of earnings in the affiliate marketing business.
Summary
In conclusion, affiliate marketing can be a rewarding venture if you understand its dynamics, select the right niche, choose the best affiliate programs and networks, build an effective marketing strategy, utilize multiple marketing channels, track and analyze your performance, overcome challenges, and learn from successful affiliate marketers. It’s a journey that requires patience, consistency, and adaptability. But with the right approach, it can lead to a significant passive income stream. So why wait? Embark on your affiliate marketing journey today and unlock your earning potential!
Frequently Asked Questions
How do I become an affiliate marketer?
To become an affiliate marketer, start by creating a website or blog, choosing a niche, researching products, and signing up for an affiliate program. Once you’ve done that, focus on building a professional website, choosing affiliate programs, creating excellent content, and networking with brands and businesses to succeed in affiliate marketing. Remember to continuously engage with your audience and strive to build trust through your content. Good luck on your affiliate marketing journey!
Can you make $10000 a month with affiliate marketing?
Yes, by employing effective tactics and strategies, individuals can achieve significant income in affiliate marketing, surpassing $10,000 per month. Aim high and implement proven strategies to reach your income goals!
Does affiliate marketing really pay?
Yes, affiliate marketing can pay, but it’s important to note that nearly 57% of affiliate marketers make less than $10,000 a year, while 16.9% make more than $20,000 annually. Keep in mind that success in affiliate marketing requires dedication and strategy.
What is affiliate marketing and how does it work?
Affiliate marketing is a performance-based strategy where individuals promote a merchant’s products for a commission. Affiliates, merchants, networks, and customers are the core players in this industry, working together to drive sales and revenue.
How do I choose the right niche for my affiliate marketing business?
Choose a niche that aligns with your passion and has sufficient demand. Understanding competition and finding unique angles within a niche can also be beneficial. Good luck with your affiliate marketing journey!